Project sector
Healthcare, IVF, Technology
Duration
8 months
(Jun 2021-Jan 2022)
Tools
Figma, Miro, Jira
Pen and Paper
My role - lead designerUser research & Synthesis, Ideation, Wireframing, Prototyping, Usability testing, Handoff to the Software team, Software testing.
Team2 Product Managers, 2 UX Designers, 3 Software Engineers, 2 Data Scientists.
Alife Health is a San Francisco-based company that is building tools to improve the equity and efficacy of IVF for everyone through artificial intelligence.
Challenge
How might we optimize the ovarian stimulation step of IVF?
The ovarian stimulation determines the success of in vitro fertilization (IVF), as physicians must carefully select the optimal medication dose, balancing cost reduction and maximizing mature egg yield. Additionally, choosing the right day for the trigger shot administration is equally important. Success in IVF hinges on efficiently navigating these decisions, emphasizing the need to optimize the ovarian stimulation step.
solution
Create an AI-powered software designed to maximize the yield of mature eggs in an ovarian stimulation cycle, enhancing the overall patient experience.
Choose the perfect
starting dose
The starting dose tool uses a machine learning algorithm to generate mature eggs retrieved predictions for a range of starting doses, enabling you to optimize the mature eggs yield (MII) and reduce medication costs.
How it works
Utilizing a patient's age and other fertility characteristics, the tool identifies 100 patient cycles with similar baseline fertility characteristics from a database of 40,000 cycles.
It uses the doses that those patients received and the corresponding outcomes of mature eggs retrieved (MII) from their cycles to generate predictions for the patient of interest.
results
12% improvement in ovarian stimulation outcomes
2000$ medication costs reduction per patient
“Since incorporating Stim Assist into my practice I have been able to use less medication and obtain more mature eggs, saving my patients money while improving their outcomes”
- Doctor C. Davis
my methodology
I structured my approach into four key steps.
Discover
User interviews
Personas
User scenarios
Flowcharts
User journey map
Define
Brainstorming
Information architecture
Sketches
Low-fi wireframes
User tests
Develop
Hi-fi wireframes
Clickable prototypes
Usability tests
Affinity maps
Design system
Deliver
Product handoff to the SW team
SW tests
Product launch
discover
Initially, we conducted interviews with reproductive endocrinologists from various clinics across the United States to gain insights into their clinical workflow and address relevant issues.
Through user interviews, we discovered that the most challenging aspects of ovarian stimulation are the initial dosage determination and trigger shot administration.
Physicians encounter numerous decisions during these crucial steps, often necessitating a second opinion based on the patient's unique case. Recognizing this need, we identified an opportunity for the development of an AI-driven support tool.
define
We developed multiple information architecture flows, explored diverse layout options through brainstorming sessions, and tested these hypotheses with users.
We incorporated valuable feedback to iteratively refine the designs, ultimately solidifying the content for the core feature set.
develop
I expanded the B2B design system to establish a cohesive visual identity and created high-fidelity wireframes.
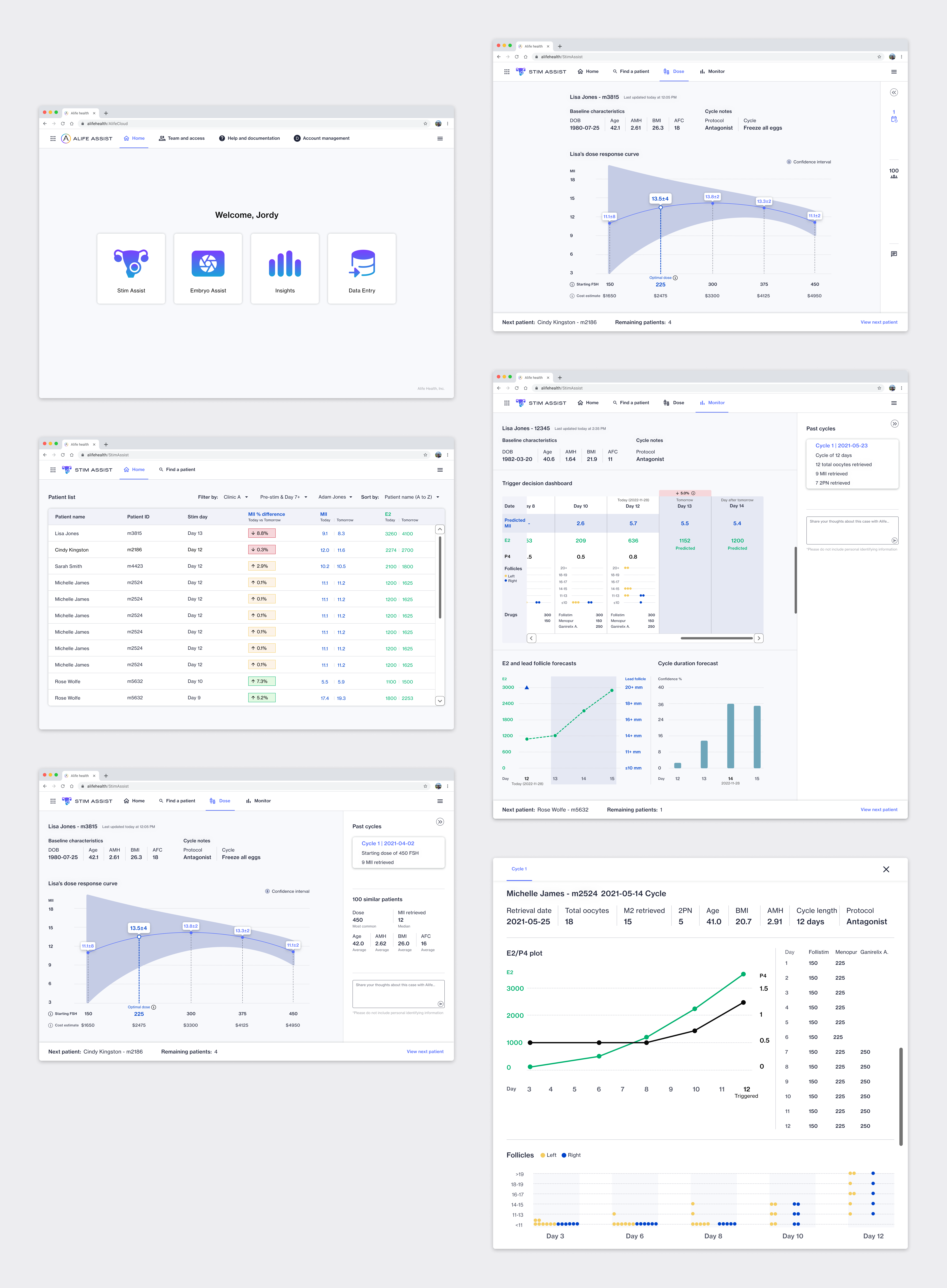
We conducted a few user test rounds to simplify visuals and interactions as I aimed to make information easily digestible for physicians accustomed to manual processes and the intricacies of outdated, crowded software.
“Stim Assist’s UI is clean, easy to use, matches my workflow well and the way it displays information is head and shoulders above any other software platform I use in my day-to-day work” - Doctor R. Jones
deliver
I collaborated closely with the software team to present and discuss the final work. Contributing to the quality assurance process, I thoroughly checked the software for bugs and ensured alignment with the wireframes before the launch. It was a team effort, and together, we successfully launched the product! 🚀
Key learnings and challenges
1. The power of design in medical complexity: I embraced the role of primary designer for this intricate data-driven ecosystem, despite my initial concerns about the complexity and my lack of medical background, and it turned out to be a highly rewarding journey.
I discovered the positive impact that we can make on both IVF patients and physicians through the power of design and collaboration, and I am grateful for the support and contributions of my colleagues throughout this impactful journey. Moving forward, I am enthusiastic about leveraging this experience in my continued pursuit of contributing to meaningful and impactful design solutions.
2. Enhancing personal hard and soft skills: The project was a practical arena for honing hard skills, such as research and visual design, and soft skills, including presentation and collaboration. Collaborating with a cross-functional team exposed me to different research methodologies and strengthened my ability to articulate and present my work effectively.